- Key Takeaways
- Mastering Predictive Customer Support: Top Strategies & Benefits
- Introduction
- Understanding Predictive Customer Support
- Key Benefits of Predictive Customer Support
- Implementing Predictive Customer Support in Your Business
- Real-World Applications of Predictive Customer Support
- Overcoming Challenges in Predictive Customer Support
- Future Trends in Predictive Customer Support
- Summary
- Call to Action
- Frequently Asked Questions
Key Takeaways
Predictive customer support utilizes advanced data analytics to anticipate and address customer needs, enhancing customer satisfaction and loyalty.
Implementing predictive analytics requires effective data management, the right tools, and continuous training for support teams to maximize its benefits.
The future of predictive customer support will be significantly influenced by advancements in AI and sentiment analysis, providing deeper insights and personalized customer interactions.
Mastering Predictive Customer Support: Top Strategies & Benefits
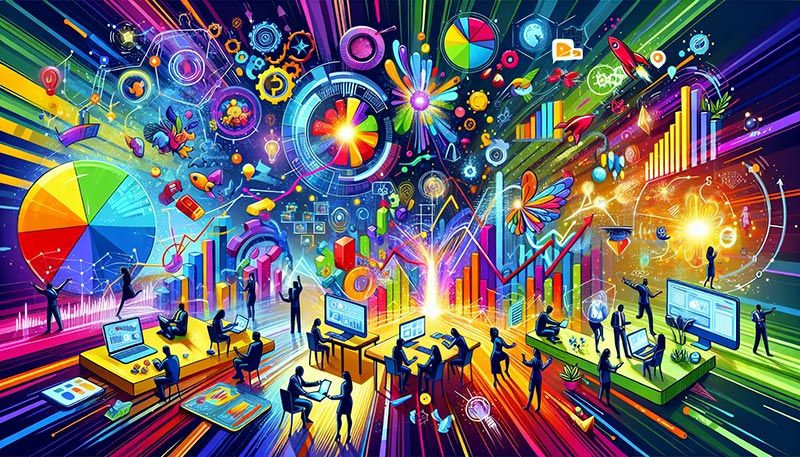
Introduction
In an age where customer experience is paramount, the role of customer support has evolved significantly. No longer is it just about resolving issues; it’s about anticipating needs and mitigating pain points before they arise. Understanding the entire customer journey, from browsing behavior to post-purchase interactions, enables businesses to create a seamless and satisfying experience through their customer service department.This shift towards predictive customer support is not just a trend but a necessity for companies looking to thrive in a competitive market.Understanding Predictive Customer Support
Predictive customer service represents a paradigm shift in how businesses approach customer service. At its core, it applies advanced data analytics to anticipate and address customer needs proactively. Analyzing vast amounts of customer data, including historical interactions and feedback, allows companies to forecast future customer behavior and tailor their support strategies.The power of predictive customer support lies in its ability to transform reactive service into proactive support. Instead of waiting for customer inquiries or complaints, support teams can use predictive analytics to identify potential issues and resolve them before they impact the customer. This leads to an enhanced customer experience, as customers feel valued and understood, knowing that their needs are being anticipated and met.Implementing predictive customer support requires a deep understanding of predictive models and how they work. These models leverage historical customer data to make accurate predictions about future behaviors and needs. Continuous analysis of customer interactions and feedback helps businesses refine these models, ensuring their accuracy and effectiveness. The result is a customer support system that not only meets but exceeds customer expectations, leading to increased customer satisfaction and loyalty.How Predictive Analytics Works in Customer Support
Predictive analytics in customer support involves using historical data to forecast future customer behavior and needs. Analyzing past customer interactions allows businesses to identify patterns and trends, providing valuable insights into future customer behavior. This enables support teams to anticipate customer issues and address them proactively, leading to fewer unhappy customers and an overall better customer support experience.Predictive models, built through machine learning algorithms, play a crucial role in this process. These models analyze vast amounts of customer data to identify patterns and make accurate predictions about future customer needs.Continuous refinement of these models with new data ensures their relevance and effectiveness, equipping support teams with the necessary insights for proactive and personalized customer service.The Role of AI in Predictive Customer Support
Artificial intelligence (AI) significantly enhances predictive customer support by automating the analysis of customer data and delivering real-time insights. AI-driven platforms can quickly process large volumes of data, providing support teams with the information they need to anticipate customer needs and respond proactively. This not only improves the efficiency of customer support operations but also leads to increased customer satisfaction as issues are resolved swiftly and effectively.AI also enables businesses to automate certain actions based on predictive insights. For example, AI can trigger automated responses to common customer inquiries or alert support agents to potential issues before they escalate.Leveraging AI in predictive customer support enables businesses to offer more personalized and proactive service, enhancing the overall customer experience and strengthening customer relationships.Key Benefits of Predictive Customer Support
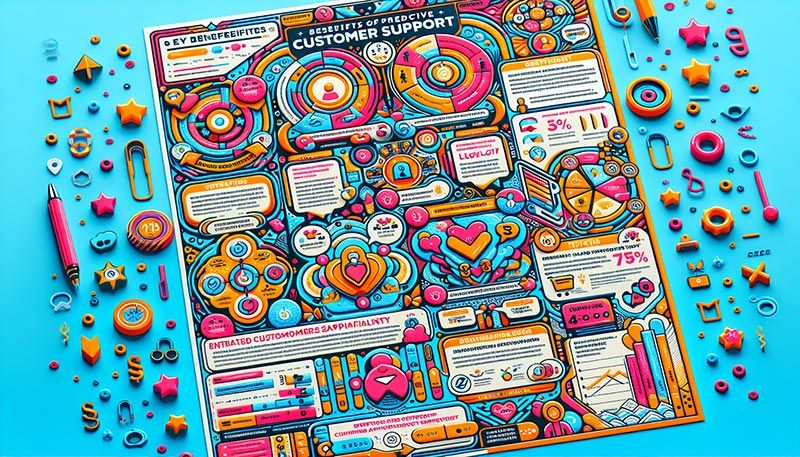
Enhanced Customer Satisfaction
Predictive customer support plays a pivotal role in enhancing customer satisfaction by enabling businesses to anticipate and address customer needs proactively. Analyzing customer data and feedback provides companies with a nuanced understanding of customer behaviors and preferences, enabling them to address issues before they escalate. This proactive approach not only reduces resolution times but also leads to fewer unhappy customers and a more satisfying customer experience.Generative AI chatbots, for example, can respond to customer inquiries instantly, vastly improving response times and handling a large volume of interactions. This not only enhances customer satisfaction but also frees up support agents to focus on more complex issues, providing better customer support overall.Personalized communication powered by predictive analytics fosters a stronger emotional connection between customers and brands, contributing to increased customer loyalty.Improved Resource Allocation
Predictive analytics aids in optimizing resource allocation by forecasting peak service times and enabling businesses to allocate staff efficiently. Understanding when demand is likely to be high or low allows companies to adjust their resources accordingly, ensuring adequate support during peak times and reducing costs during slower periods.This effective resource allocation leads to shorter wait times for customers, improving overall customer satisfaction.Increased Customer Loyalty
Personalizing customer interactions through predictive analytics builds deeper, more meaningful relationships with customers, fostering loyalty and reducing the risk of churn.Predictive analytics also enables businesses to implement targeted retention strategies, minimizing customer churn and strengthening customer loyalty over time. This combination of personalized interactions and effective churn management contributes to a loyal customer base and long-term business success.Implementing Predictive Customer Support in Your Business
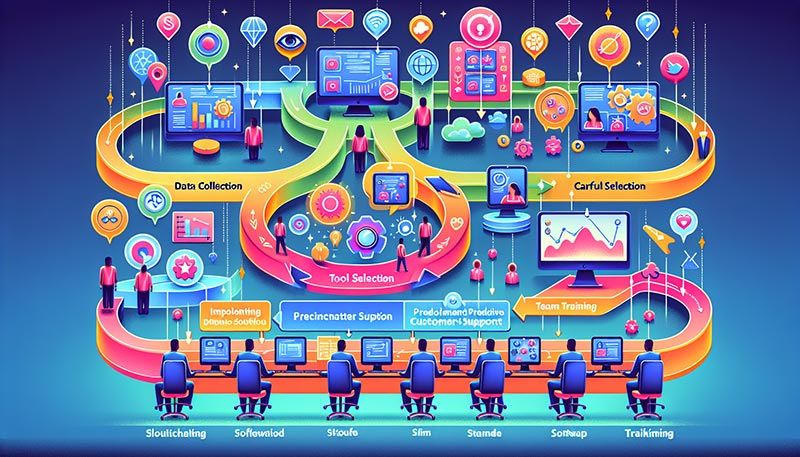
Data Collection and Management
Effective data management is the backbone of successful predictive customer support. This involves gathering diverse customer data points, such as transaction histories, demographics, and behavior patterns, to boost predictive accuracy. Ensuring data is clean and accurately organized is crucial for effective integration and analysis. Regular audits of predictive models help identify and mitigate biases, ensuring the insights generated are fair and reliable.To maintain the quality and reliability of data, businesses should implement continuous monitoring and regular evaluations of their predictive models. This ensures that any emerging biases are identified and corrected promptly, maintaining the fairness and accuracy of predictions.By managing data effectively, businesses can gain valuable insights into customer behaviors and preferences, enabling them to anticipate customer needs and provide better support.Choosing the Right Tools and Platforms
Selecting the right tools and platforms is critical for the success of predictive customer support initiatives. Businesses should focus on tools that can integrate seamlessly with their existing systems, ensuring a smooth transition and optimal performance. It’s important to assess the functionality and ease of use of these tools to ensure they meet the specific needs of the business. Recent advancements in AI and machine learning technologies have enabled more personalized and efficient customer support experiences, making it essential to stay updated with the latest developments.When choosing predictive analytics tools, businesses should consider their ability to provide accurate predictions and valuable insights into customer behavior. This helps in making informed decisions and optimizing support strategies.By selecting the right tools and platforms, businesses can enhance their predictive customer support capabilities, leading to improved customer satisfaction and loyalty.Training and Development for Support Teams
Continuous training and development for support teams are vital for maximizing the benefits of predictive customer support. Support agents need to be well-versed in using predictive analytics tools and interpreting data insights to provide proactive and personalized customer service. Ongoing training ensures that support teams stay updated on the latest trends and techniques in predictive analytics, enabling them to handle customer interactions more effectively.Development programs should focus on enhancing the analytical skills of support teams and familiarizing them with the functionalities of predictive tools. By investing in continuous training, businesses can ensure that their support teams are equipped to leverage predictive insights, leading to an enhanced customer experience and better customer support overall.Real-World Applications of Predictive Customer Support
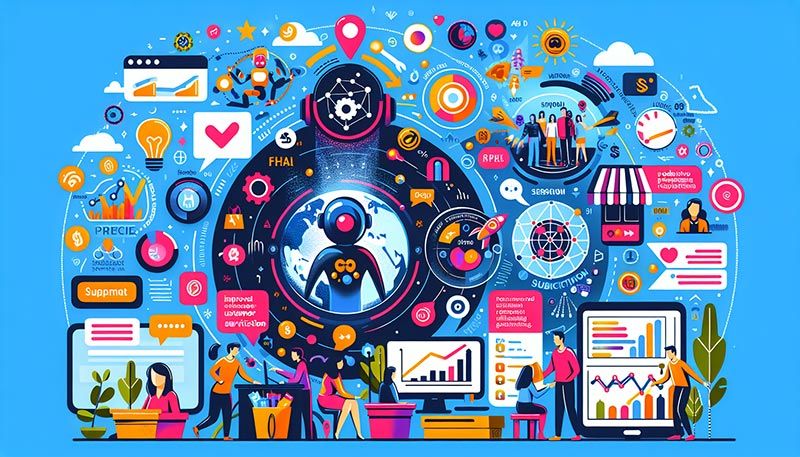
Case Study: Reducing Customer Churn
Delta Air Lines leverages predictive analytics to forecast customer demand and predict the required number of support agents, which helps in identifying at-risk customers. Employing proactive measures enabled by predictive insights allows Delta to adjust its strategies and resources, leading to a notable improvement in customer satisfaction scores.This case study highlights the effectiveness of predictive customer support in reducing churn and enhancing customer loyalty.Case Study: Enhancing Personalization
Netflix utilizes predictive analytics to analyze viewing history and recommend shows and movies tailored to individual preferences. This personalized approach significantly enhances customer satisfaction, as users receive recommendations that align with their interests.Similarly, Missouri Star Quilt Co. uses predictive analytics to identify likely purchasers and offer personalized recommendations, resulting in a 47% increase in average order value. These examples underscore the power of predictive customer support in delivering personalized experiences and increasing customer satisfaction.Overcoming Challenges in Predictive Customer Support
Implementing predictive customer support comes with its own set of challenges, but understanding and addressing these can ensure a smoother transition and more effective outcomes. One of the primary challenges is ensuring data privacy and security. With increasing public scrutiny, companies must take data privacy seriously by limiting access and using data masking techniques to protect sensitive customer information.Managing bias in predictive models is another significant challenge. Bias can lead to unfair treatment of certain groups and inaccurate predictions. Organizations should adopt strategies such as diversifying training data, adjusting algorithm parameters, and implementing bias detection tools to ensure fairness and accuracy in their predictive analytics. Continuous monitoring and evaluation of predictive models are crucial to identify and correct any emerging biases over time.Integrating predictive analytics tools with existing systems can be technically challenging, often requiring thorough planning and collaboration between IT and business units. Legacy systems may need updates or middleware solutions to ensure compatibility with newer technologies. Successful integration involves seamless collaboration and strategic planning to ensure that predictive analytics tools enhance the overall customer support experience.Ensuring Data Privacy and Security
In the realm of predictive customer support, respecting data privacy and ensuring the security of customer data are paramount. Effective data management practices are crucial for maintaining the quality and reliability of data used in predictive analytics.Public scrutiny has led companies to take data privacy more seriously, implementing measures such as data masking and limiting access to sensitive information to protect customer data and maintain trust.Managing Bias in Predictive Models
Bias in predictive models can result in inaccurate predictions and negative business outcomes. To ensure fairness, organizations should diversify their training data, adjust algorithm parameters, and implement bias detection tools.Continuous monitoring and evaluation of predictive models are essential to identify and correct any biases that may emerge over time, ensuring accurate and fair predictions.Integrating with Existing Systems
Seamless integration of predictive analytics tools with existing systems is often hampered by technical challenges. Successful integration requires thorough planning and collaboration between IT and business units, as well as potential updates to legacy systems to ensure compatibility with newer technologies.By addressing these challenges, businesses can enhance their customer support capabilities and provide a more seamless and satisfying customer experience to improve customer satisfaction.Future Trends in Predictive Customer Support
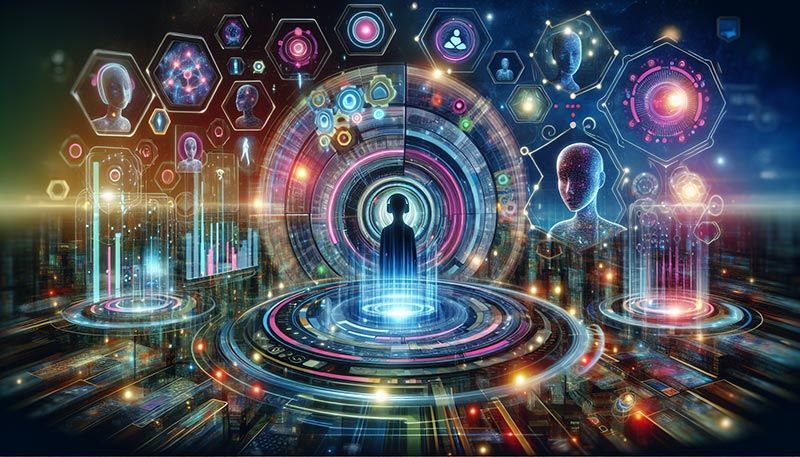